안녕하세요. 이번 포스팅은 ViT 코드 구현을 해보려고 합니다.
ViT에 대해서는 Transformer 포스팅에서 살짝 언급했었는데요, ViT는 이제 많은 vision task의 backbone으로 쓰이고 있습니다.
[Transformer] Transformer & Vision
안녕하세요. 이번 ML관련 포스팅에서는 Transformer관련하여 포스팅하겠습니다. 이미 나온지 꽤 오래되었고 많은 분야에서 활용되고 있는 아키텍쳐인데요. NLP분야에서 일찍이 탄생했지만 비전 및
jaehoon-daddy.tistory.com
위의 대략적인 overview를 보면 image를 patch(or token)로 나누고 position encoding과 summation후에 Transformer Encoder를 거치게 됩니다.
그럼 우선 Image data를 patch로 나누는 코드를 살펴보겠습니다.
def generate_patch(patch_size, image):
num_channels = image.size(0) # Dataloader거친 후 Batch_size, Channel, W, H
patches_w = image.unfold(
1, patch_size, patch_size
) # dimension, size, step, w기준으로 patch나누는과정
patches = patches_w.unfold(2, patch_size, patch_size) # h기준으로 patch화
patches = patches.reshape(
num_channels, -1, patch_size, patch_size
) # 3, patch개수 , patchsize_w, patchsize_h
patches = patches.permute(
1, 0, 2, 3
).contiguous() # patch개수, 3, patchsize_w, patchsize_h
flatten_patches = patches.reshape(
patches.size(0), -1
) # patch개수, 3 x patch_w x patch_h
return flatten_patches
def __getitem__(self, index):
if self.training:
input_data = self.train_set[index]
label = self.labels[index]
else:
input_data = self.test_set[index]
label = None
if self.cfg.PATCH:
patched_input_data = generate_patch(self.cfg.PATCH.PATCH_SIZE, input_data)
return patched_input_data, label
__getitem__에서 input_data인 image데이터와( W x H x C )와 config파일에서 patch_size를 인자로 하여 generate_patch로 넘겨줍니다. 실제로는 generate_patch에서 patch화가 진행됩니다. patch로 나눌때 unfold라는 torch 기능을 사용합니다. (1,patch_size,patch_size)는 w방향으로 patch_size로 patch_size의 step으로 split하는 걸 의미합니다. patches_w.unfold(2, patch_size, patch_size) 단계를 거치면 x,y방향으로 모두 patch화를하게 되고 이를 처음의 channel을 기준으로 다시 reshape 하게 됩니다. 이를 최종 (patch개수, 채널수 x patch_w x patch_h)의 형태로 flatten합니다.
*view, reshape, transpose, permute의 차이
view. vs reshape
x = torch.rand(2, 3, 4) # [2, 3, 4]
y = x.view(2, -1) # [2, 12]
x = torch.rand(2, 3, 4) # [2, 3, 4]
x = x.reshape(2, -1) # [2, 12]
우선 view와 reshape은 동일한 기능을 합니다. 차이는 view는 pointer처럼 수정시 원본 data도 변경이 되고 reshape은 copy 혹은 pointer를 반환한다고 합니다.(어떤걸 받을지는 모른다는 이야기)
permute vs transpose
transpose는 두차원을 서로 맞교환할 수 있고, permute는 모든 차원을 맞교환 할 수 있습니다.
x = torch.rand(16, 32, 3)
y = x.tranpose(0, 2) # [3, 32, 16]
z = x.permute(2, 1, 0) # [3, 32, 16]
또한 permute, transpose 둘다 모두 view와 같이 원본 data를 공유하는 포인터로 활용된다. 또한 permute, transpose와 view의 차이점은 view는 contiguous tensor에서만 작용할 수 있다는 것 입니다. 예를 들면 transpose를 contiguous하게 하려면 transpose().contiguous()를 불러와야 합니다. reshape은 contiguous와 상관없이 사용할 수 있습니다.
Contiguous란 메모리에 연속적인 array라는 뜻입니다. n차원의 array도 추상화되어있을 뿐 물리적으로는 일렬의 메모리에 저장되어있습니다.즉 coutiguous란 array에서 물리적으로 행방향으로 연속된 형태를 의미합니다.
다음으로 overview상의 linear projection을 보겠습니다.
generate_patch함수를 거쳐서 나온 patch data가 batch형태로 (patch개수, 3 x patch_w x patch_h) input으로 들어가게됩니다.
class EmbeddingMudule(nn.Module):
def __init__(
self, patch_vector_size, patches_num, latent_vector_dimension, drop_rate=0.1
):
super(EmbeddingMudule, self).__init__()
self.linear_projection = nn.Linear(patch_vector_size, latent_vector_dimension)
self.class_token = nn.Parameter(torch.randn(1, latent_vector_dimension))
self.positional_emdedding = nn.Parameter(
torch.randn(1, patches_num + 1, latent_vector_dimension)
)
self.dropout = nn.Dropout(drop_rate)
def forward(self, x):
# x : B, patch개수, 3 x patch_w x patch_h
# repeat == expand
# linear_proj : B, patch개수, latent_vector_dimension, cls_token : B, 1, 1
# B, patch개수 + 1, latent_vector_dimension
batch_size = x.size(0)
x = torch.cat(
[self.class_token.repeat(batch_size, 1, 1), self.linear_projection(x)],
dim=1,
)
# B, patch개수 + 1, latent_vector_dimension
x += self.positional_emdedding
x = self.dropout(x)
return x
x = torch.cat([self.cls_token.repeat(batch_size, 1, 1), self.linear_proj(x)], dim=1) 이 부분을 보면 input_data를linear_projection에 넣어 latent_vector_dimentsion으로 shape을 변경함과 동시에 mlp를 한번 태웁니다. class_token의 경우 랜덤하게 latent_vector_dimension만큼 파라미터를 생성한 후에 (batch_size, 1, latent_vector_dimentsion)로 shape을 expand하고 linear_projection의 (B, patch개수, latent_vector_dimension)과 concat하여 최종적으로 (B, patch개수+1, latent_vector_dimension)으로 shape이 만들어집니다.
이 후 positional_emdedding과정을 거칩니다. position encoding방법은 여러가지가 있고 보통 cos,sin 파형을 이용해서 하는데 여기서는 learnable parameter를 추가하는걸로 embedding을 진행하였습니다. (B, patch개수+1, latent_vector_dimension) 형식의 leanable parameter를 만든후에 이를 summation하였습니다.
*repeat, expand 차이
repeat의 경우 특정 tensor의 size 차원의 데이터를 반복합니다.
>>> x = torch.tensor([1, 2, 3])
>>> x.repeat(4, 2)
tensor([[ 1, 2, 3, 1, 2, 3],
[ 1, 2, 3, 1, 2, 3],
[ 1, 2, 3, 1, 2, 3],
[ 1, 2, 3, 1, 2, 3]])
>>> x.repeat(4, 2, 1).size()
torch.Size([4, 2, 3])
예를 들면 [1,2,3]을 dim=0으로 4만큼 dim=1로 2만큼 반복하면, (4, 6)의 차원이 나오게 됩니다. 그리고 repeat은 원래의 data를 copy합니다.
expand의 경우는 마찬가지로 특정 tensor를 반복하여 생성하는데 개수가 1인 차원만 적용할 수 있습니다.
>>> x = torch.tensor([[1], [2], [3]])
>>> x.size()
torch.Size([3, 1])
>>> x.expand(3, 4)
tensor([[ 1, 1, 1, 1],
[ 2, 2, 2, 2],
[ 3, 3, 3, 3]])
>>> x.expand(-1, 4) # -1 means not changing the size of that dimension
tensor([[ 1, 1, 1, 1],
[ 2, 2, 2, 2],
[ 3, 3, 3, 3]])
expand는 원본을 참조합니다.
다음단계는 Transformer Encoding입니다.
class TransformerEncoder(nn.Module):
def __init__(self, latent_vector_dim, head_num, mlp_hidden_dim, drop_rate=0.1):
super().__init__()
self.ln1 = nn.LayerNorm(latent_vector_dim)
self.ln2 = nn.LayerNorm(latent_vector_dim)
self.msa = MultiheadedSelfAttention(
latent_vector_dim=latent_vector_dim,
head_num=head_num,
drop_rate=drop_rate,
)
self.dropout = nn.Dropout(drop_rate)
self.mlp = nn.Sequential(
nn.Linear(latent_vector_dim, mlp_hidden_dim),
nn.GELU(),
nn.Dropout(drop_rate),
nn.Linear(mlp_hidden_dim, latent_vector_dim),
nn.Dropout(drop_rate),
)
def forward(self, x):
# B, patch개수 + 1, latent_vector_dim
z = self.ln1(x) # B, patch개수 + 1, latent_vector_dim
z, attention_vector = self.msa(
z
) # z : B, patch개수 + 1 ,latent_vector_dim(head_number x head_dimension)
z = self.dropout(z)
x = x + z
z = self.ln2(x)
z = self.mlp(z)
x = x + z # B, patch개수 + 1 ,latent_vector_dim
return x, attention_vector
Embedding 모듈 이후에 (B, patch개수 + 1, latent_vector_dim)의 shape의 data를 normalization을 태우고나서 multi-head-attention과정을 거치게 됩니다. mha은 아래 자세히 설명하겠습니다. 이 후 다시 normalization과정과 mlp과정을 거치고 나서 residual block처럼 원래의 input feature를 summation하는 것이 transformer encoding과정의 전부입니다.
*layer norm vs batch norm
layer norm은 batch에 어떤 크기의 데이터들이 있든 관계없이 샘플데이터 단위로 normalization을 시켜줍니다. batch norm은 mini-batch내부 feature값들의 mean,std로 normalization을 진행합니다.
class MultiheadedSelfAttention(nn.Module):
def __init__(self, latent_vector_dim, head_num, drop_rate, device):
super().__init__()
self.device = device
self.head_num = head_num
self.latent_vector_dim = latent_vector_dim
self.head_dim = int(latent_vector_dim / head_num)
self.query = nn.Linear(latent_vector_dim, latent_vector_dim)
self.key = nn.Linear(latent_vector_dim, latent_vector_dim)
self.value = nn.Linear(latent_vector_dim, latent_vector_dim)
self.dropout = nn.Dropout(drop_rate)
def forward(self, x):
# B, patch개수 + 1, latent_vector_dim
batch_size = x.size(0)
q = self.query(x) # q : B, patch개수 + 1, latent_vector_dim
k = self.key(x) # k : B, patch개수 + 1, latent_vector_dim
v = self.value(x) # v : B, patch개수 + 1, latent_vector_dim
q = q.view(batch_size, -1, self.head_num, self.head_dim).permute(
0, 2, 1, 3
) # B, patch개수 + 1 ,head number, head dimension -> B, head_number, patch개수 + 1, head_dimension(int(latent_vector_dim / num_heads)
k = k.view(batch_size, -1, self.head_num, self.head_dim).permute(
0, 2, 3, 1
) # B, patch개수 + 1 ,head number, head dimension -> B, head_number, head_dimension(int(latent_vector_dim / num_heads), patch개수 + 1
v = v.view(batch_size, -1, self.head_num, self.head_dim).permute(
0, 2, 1, 3
) # B, patch개수 + 1 ,head number, head dimension -> B, head_number, patch개수 + 1, head_dimension(int(latent_vector_dim / num_heads)
attention = torch.softmax(
q @ k / torch.sqrt(self.head_dim * torch.ones(1)), dim=-1
) # B, head_number, patch개수 + 1, patch개수 + 1
x = (
self.dropout(attention) @ v
) # B, head_number, patch개수 + 1, patch개수 + 1 x B, head_number, patch개수 + 1, head_dimension => B, head_number, patch개수 + 1, head_dimension
x = x.permute(0, 2, 1, 3).reshape(
batch_size, -1, self.latent_vector_dim
) # B, patch개수 + 1, head_number, head_dimension -> B, patch개수 + 1 ,latent_vector_dim(head_number x head_dimension)
return x, attention
앞서 transformer encoder에서 msa로 (B, patch개수 + 1, latent_vector_dim) shape의 normalized된 data가 input으로 들어가는 것을 봤습니다.
이제 그림과 같은 self-attention의 과정을 거치게 됩니다. input data을 각각 learnable parameter와의 linear layer를 태워서 동일한 shape의 (B, patch개수 + 1, latent_vector_dim)를 query, key, value vector를 생성하고 최종적으로 아래와 같은 shape을 만듭니다.
q : B, head_number, patch개수 + 1, head_dimension
k : B, head_number, head_dimension, patch개수 + 1
v : B, head_number, patch개수 + 1, head_dimension
이 후 softmax(q,kT / root(D))*v 을 계산하여 최종적으로 B, patch개수 + 1, head_number, head_dimension 가 나오게 되고 이를 다시 B, patch개수 + 1 ,latent_vector_dim(head_number x head_dimension) 형태로 reshape합니다.
처음의 shape과 동일합니다. self-attention은 input shape과 동일한 모습입니다.
mlp 헤드 단계입니다. transformer encoder에서 나온 output을 단순히 norm과 linear layer를 태우는 것이 전부입니다. 그래서 최종적으로는 class만큼의 layer가 output shape으로 나오게 됩니다.
self.mlp_head = nn.Sequential(
nn.LayerNorm(latent_vector_dim), nn.Linear(latent_vector_dim, num_classes)
)
여기까지 ViT코드 설명이였습니다. 전체 코드는 아래 github에서 볼 수 있습니다.
GitHub - ies0411/openViT: PyTorch Implementation of "ViT", with Korean Comments
PyTorch Implementation of "ViT", with Korean Comments - GitHub - ies0411/openViT: PyTorch Implementation of "ViT", with Korean Comments
github.com
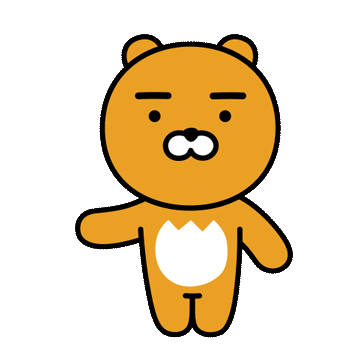